Research Phase 3
Predictive Quality and Data-Fusion in Ultra-Short-Pulsed Laser Structuring
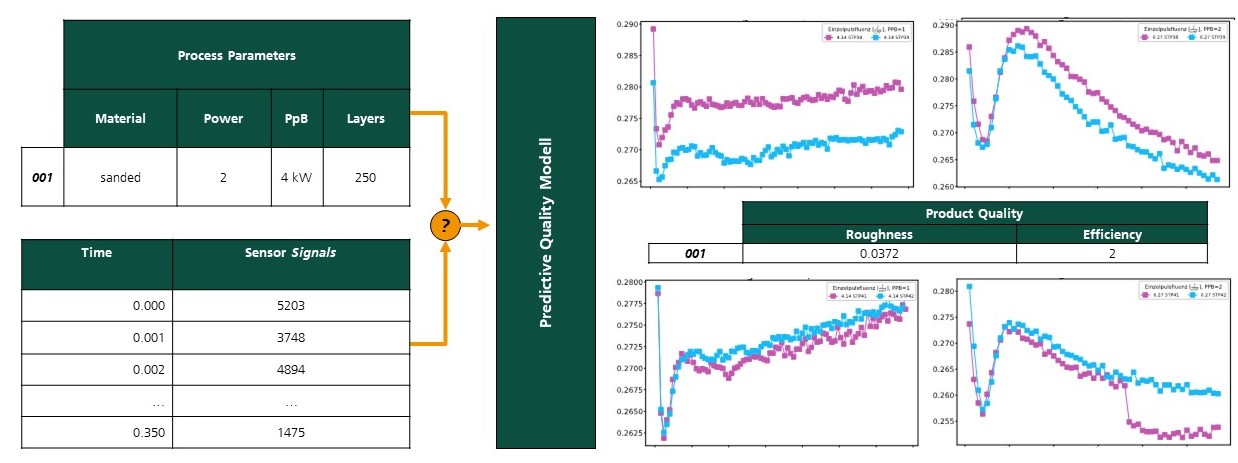
The realization of nanostructures by ultra-short-pulsed laser structuring allows the precise modification of visual, haptic, and functional surface properties for various materials. Due to its precision and unlimited freedom of design, completely new component properties can be achieved, especially in high-tech applications such as toolmaking or medical technology. However, regarding growing sustainability concerns, long process duration and high energy consumption are disadvantages.
Machine learning has the potential to fit accurate process models utilizing process data, laying the foundation for process optimization that addresses the aforementioned drawbacks. However, in laser structuring, the information is distributed over a variety of data sources that generate data of various modalities (i.e., process parameters as cross-sectional data and process monitoring data as time-series measurements). The main challenge for data-driven process optimization for laser structuring, and for the vast majority of production processes in general, is the fusion of process parameters and process monitoring data.
Therefore, in this project we investigated which approaches can be used to extract information from process monitoring and integrate it into process parameters to train predictive quality models for laser structuring. The results show that the trained models can very accurately and reliably predict the surface properties of the structured components and that the integration of process monitoring further improves prediction accuracy. The evaluation over different initial surface properties confirms that the models exhibit a compliant behavior: For example, the influence of process monitoring increases significantly for polished components compared to milled components, since the influence of the process is significantly greater with smooth surfaces. The approach from this project can also be applies to other industry project with comparable process and data characteristics.